60 Data, Analytics, and AI Terms Simplified: A Comprehensive Guide to Industry Jargon
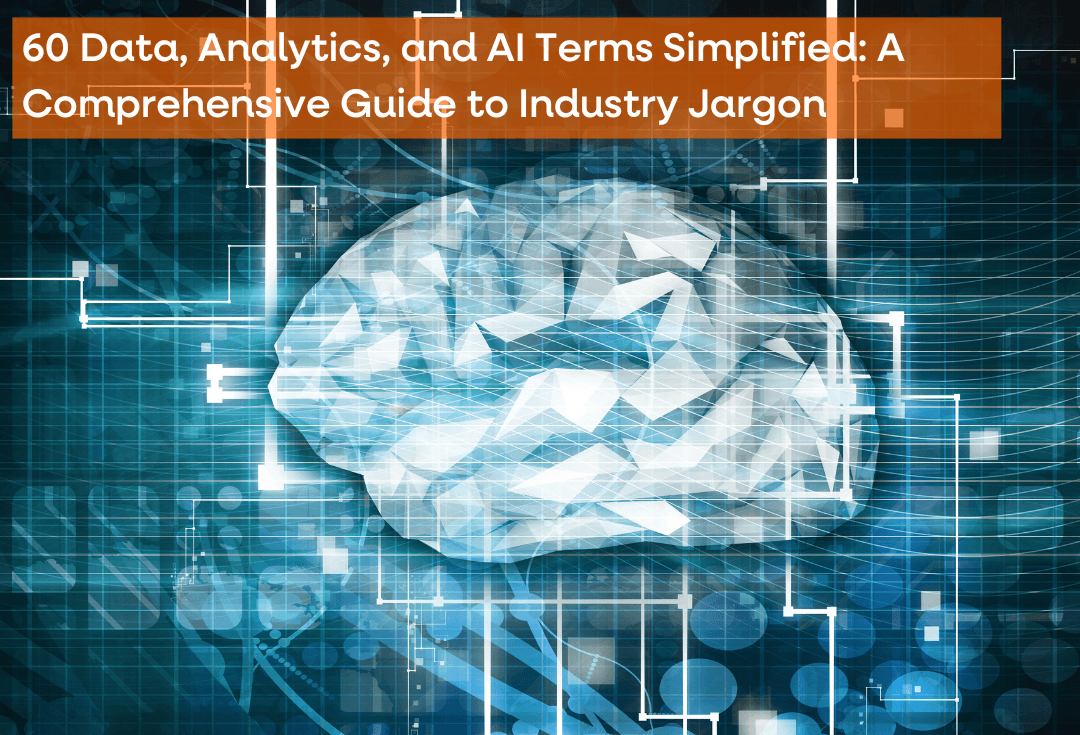
In an era dominated by data, analytics, and artificial intelligence (AI), understanding the language of these cutting-edge fields has become more crucial than ever. Whether you’re a seasoned professional navigating the intricate landscapes of data science or a newcomer curious about the terminology shaping the technological landscape, a firm grasp of the industry jargon is essential. The world is rapidly evolving, and businesses across various sectors are harnessing the power of data and AI to make informed decisions, gain competitive advantages, and drive innovation.
As more and more organizations increase their adoption of data, analytics, and AI, it’s imperative to break down the barriers of complexity that often accompany these terms. This comprehensive guide aims to demystify the vocabulary surrounding data analysis, data science, AI, machine learning, and more. Whether you’re a student, a professional looking to enhance your skills, or an enthusiast eager to explore the fascinating world of technology, this guide is your gateway to understanding the essential terms that shape the future.
- AI Ethics: Considerations and practices to ensure responsible AI development and use.
- Algorithm: A set of instructions or rules for specific tasks, used in data analysis, machine learning, and AI.
- Application Programming Interface (API): Protocols determining how software applications interact.
- Artificial Intelligence (AI): The simulation of human intelligence processes by machines or computer systems.
- Attribute: A descriptor used to label a column in a spreadsheet or database.
- Big Data: A large collection of data characterized by volume, velocity, and variety.
- Business Intelligence (BI): Strategies, technologies and Data analytics empowering data-driven business decisions.
- Chatbot: A software application imitating human conversation through text or voice commands.
- Clean Data: Accurate, complete, and analysis-ready data that undergoes data cleaning to ensure accuracy.
- Conversational AI: AI systems enabling natural language interactions between humans and machines.
- CSV (Comma-Separated Values) File: A text file format for storing data, commonly used for compatibility with spreadsheet and database software.
- Dashboard: A tool for monitoring and displaying live data with visualizations connected to databases.
- Data Analytics: The collection, transformation, and organization of data to draw conclusions, make predictions, and drive decision-making.
- Data Architecture: The plan for an organization’s data management system, covering data lifecycle touchpoints.
- Data Cleaning: The process of preparing raw data for analysis by ensuring accuracy, completeness, consistency, and lack of bias.
- Data Engineering: Making data accessible for analysis by building systems to collect, manage, and convert raw data.
- Data Enrichment: Adding data to an existing dataset during the data transformation process for better analysis.
- Data Governance: The formal plan for managing company data, including rules for access, use, accountability, and compliance.
- Data Integrity: Ensuring the accuracy, reliability, and consistency of data over time.
- Data Labels: Text elements providing specific values associated with data points in a chart.
- Data Lake: A repository for storing large amounts of structured, semi-structured, and unstructured raw data.
- Data Mart: A subset of a data warehouse focused on specific business functions.
- Data Mining: Closely examining data to identify patterns and gain insights, a central aspect of data analytics.
- Data Modeling: Creating a visual representation of the structure and relationships within a dataset, including entities, attributes, and relationships.
- Data Pipeline: A set of processes and tools that enable the automated flow of data from various sources to a destination, often involving transformations and analytics for insights or AI model training.
- Data Privacy: The protection of sensitive information from unauthorized access, ensuring that personal and confidential data is handled and managed securely, adhering to legal and ethical standards.
- Data Science: The scientific study of data involving capturing, transforming, analyzing, and creating predictive models.
- Data Source: The origin of specific information used for measuring business success and making strategic recommendations.
- Data Visualization: Representing information and data through charts, graphs, maps, and visual tools.
- Data Warehouse: A centralized repository storing processed, organized data from multiple sources.
- Data Wrangling: Converting raw data into a usable form, involving discovery, transformation, validation, and publishing.
- Database: An organized collection of information searchable, sortable, and updatable, often managed by a database management system (DBMS).
- Deep Learning: A subfield using artificial neural networks to model and solve complex problems.
- Descriptive Analytics: Focuses on summarizing and presenting historical data to describe what has happened in the past. It involves organizing and summarizing data to gain insights into patterns, trends, and key features.
- Diagnostic Analytics: Aims to understand why certain events or outcomes occurred by analyzing historical data. It involves investigating the causes of specific patterns or trends identified through descriptive analytics.
- Feature Engineering: Selecting, transforming, and creating relevant features from raw data to improve ML (Machine Learning) model performance.
- Generative AI: Technology using AI to create content, including text, video, code, and images.
- Large Language Model: An advanced AI system characterized by its extensive size, equipped with the capability to process and understand human language.
- Language Model: A type of artificial intelligence (AI) model that is trained to understand and generate human language. It is designed to predict the likelihood of a sequence of words or characters given a context.
- Machine Learning: Subset of AI where algorithms mimic human learning, improving predictions and performance over time.
- Metadata: Data about data, describing various characteristics such as collection method, storage, file type, or creation date.
- Natural Language Processing (NLP): AI enabling computers to understand spoken and written human language.
- Neural Network: A deep learning technique resembling the human brain’s structure, composed of interconnected nodes organized into layers, and excels at learning patterns from data.
- Normalization: Scaling data to a standard range for fair comparisons.
- Open Data: Publicly available data for anyone to use, useful for practicing data analysis skills.
- OpenAI: AI research organization developing advanced AI models and technologies.
- Predictive Analytics: Using technology to predict future outcomes based on historical data and patterns.
- Prescriptive Analytics: Using technology to analyze data for better strategic decisions. It goes beyond descriptive and predictive analytics by not only providing insights into what might happen but also suggesting the best course of action to achieve desired outcomes.
- Prompt: Input given to a chatbot or language model for generating a response.
- Qualitative Data: Non-numeric data describing qualities or characteristics.
- Quantitative Data: Objective, numeric data that can be counted or measured.
- Query: A request for information formulated using query languages like SQL in data analytics.
- Regression Analysis: A statistical technique modeling relationships between variables.
- Relational Database: A database with multiple tables containing related information accessible through single queries.
- Structured Data: Formatted data organized into rows and columns.
- Structured Query Language (SQL): A programming language for managing relational databases.
- Supervised Learning: A Machine learning model, trained on a labeled dataset, where both input data and corresponding output (target) are provided. The algorithm learns to map the input data to the correct output by generalizing patterns from the labeled examples.
- Training Data: Information given to an AI system to learn, find patterns, and create new content.
- Unstructured Data: Data not organized in an apparent way, requiring organization for analysis.
- Unsupervised Learning: A machine learning model trained on an unlabeled dataset where the algorithm explores and identifies patterns or structures within the data without explicit guidance.
At Synogize, we recognize the transformative power that knowledge holds in today’s digital age. As a data, analytics, and AI consulting firm, we are not only committed to staying at the forefront of technological advancements but also to sharing our expertise with you. Our dedication to promoting data literacy stems from the belief that informed individuals and businesses contribute to a more innovative and resilient society.
By delving into the intricacies of data, analytics, and AI through this comprehensive guide, we aim to empower you with the tools needed to navigate the complexities of the digital landscape. Synogize is dedicated to fostering a culture of continuous learning, providing resources, and offering consulting services to help you harness the full potential of data and AI.