Simplifying Data Analysis: The Process, Types, and Most Common Techniques.
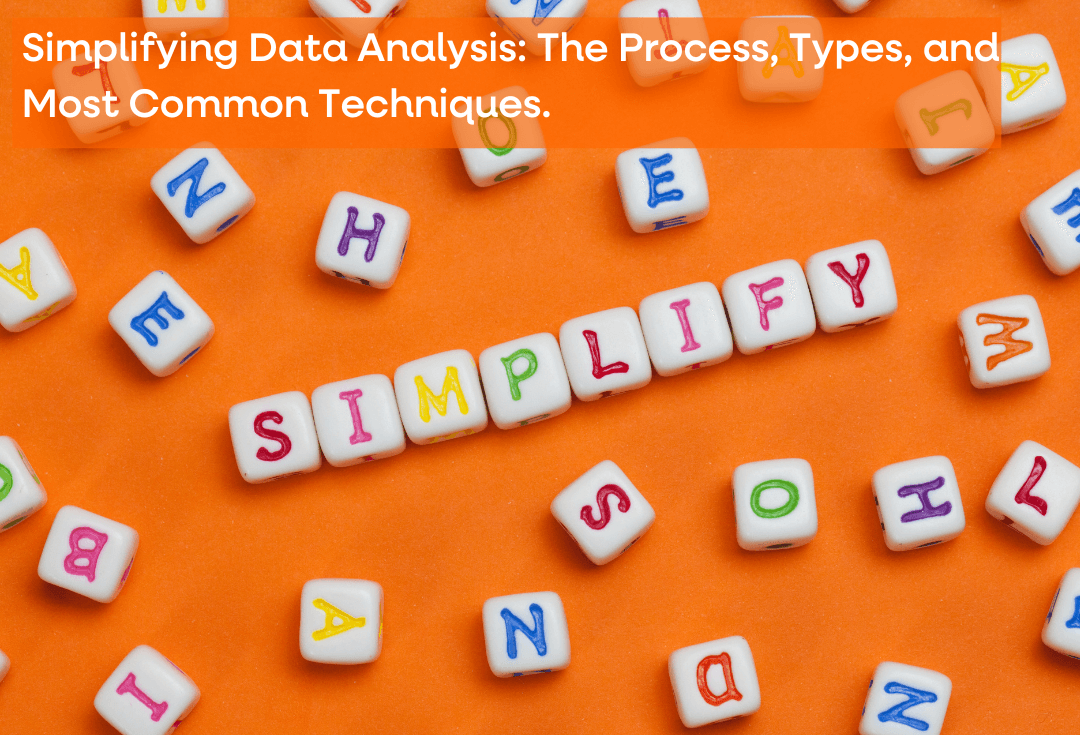
In the fast-paced digital landscape of today, data is not just an asset; it’s a powerhouse that fuels informed decision-making and strategic planning. Welcome to the world of data analysis, where raw information transforms into actionable insights, propelling businesses toward growth, efficiency, and innovation. In this blog, we’ll delve into the process of data analysis and explore its various types, shedding light on the techniques that drive this transformative journey.
Understanding Data Analysis
What is Data Analysis?
Data analysis is a systematic method encompassing inspection, cleansing, transformation, and modeling of data. Its primary goal is to unveil meaningful information, draw conclusions, and support decision-making. This multifaceted process is instrumental for organizations, providing the backbone for strategic planning across various sectors, including businesses, governments, and more.
The Importance of Data Analysis in Today’s Digital World
In the era of digital transformation, the explosion of data has led to the advent of ‘big data,’ making data analysis more critical than ever. Properly analyzed data can revolutionize businesses by offering invaluable insights that enhance products, streamline operations, and boost profitability. Consider the impact in industries such as healthcare, finance, and e-commerce, where predictive and prescriptive analyses reshape the landscape.
The Data Analysis Process: A Step-by-Step Guide
Step 1: Defining Objectives and Questions
The journey starts with a clear definition of objectives and formulating specific questions that the analysis seeks to answer. Understanding the problem, identifying necessary data, and defining metrics set the tone for the entire process.
Step 2: Data Collection
With objectives in place, relevant data is collected through surveys, interviews, observations, or existing databases. Data can be quantitative or qualitative, depending on the problem at hand.
Step 3: Data Cleaning
Data cleaning, or data cleansing, is a crucial step to ensure data quality. Errors and inconsistencies are corrected or removed, laying the foundation for accurate and meaningful results.
Step 4: Data Analysis
The cleaned data undergoes analysis using statistical or mathematical techniques. Tools like Python, R, Excel, and specialized software extract patterns, relationships, or trends.
Step 5: Data Interpretation and Visualization
Analysis results are interpreted and visualized through charts or graphs, making complex data comprehensible. Visualization aids in presenting findings clearly and concisely.
Step 6: Conveying Insights
The final step involves presenting analysis findings in a narrative form that is engaging and easy to understand. Effectively conveying insights is essential for reaching non-technical audiences and facilitating data-driven decisions.
What are the 4 The Types of Data Analysis?
Embarking on the journey of data analysis involves navigating through various types, each serving a distinct purpose in unravelling insights from raw information. The four primary types are as follows:
- Descriptive Analysis: Describes or summarizes raw data to identify patterns and trends over time. It’s the foundation, answering the question, “What happened?”For instance, in understanding sales patterns, descriptive analysis would provide a comprehensive overview of monthly sales figures, highlighting peak periods and identifying potential fluctuations.
- Diagnostic Analysis: Dives deeper into the data to determine “Why something happened”. It explores correlations, uncovering causal relationships and patterns.In the context of sales, this type might explore correlations between marketing strategies and sales spikes, uncovering causal relationships and patterns that explain variations in performance.
- Predictive Analysis: Utilizes statistical models and forecasting techniques to predict future outcomes based on historical data – “What will happen”. Common applications include risk assessment and sales forecasting.In the sales scenario, predictive analysis could forecast future sales trends, allowing businesses to proactively adjust inventory levels or marketing strategies to meet anticipated demand.
- Prescriptive Analysis: Representing the pinnacle of data analysis, prescriptive analysis not only predicts outcomes but also suggests actions to capitalize on those predictions. Leveraging technologies like AI, it goes beyond forecasting to recommend decisions that can drive optimal results “How can we make it happen”.Continuing with the sales example, prescriptive analysis might suggest specific marketing campaigns or pricing strategies based on predicted sales trends, guiding decision-makers towards optimal results.
In essence, this comprehensive framework equips analysts to extract valuable insights at each stage of the data analysis process, providing actionable information for informed decision-making in various domains, including sales and beyond.
Most Common Data Analysis Techniques
The four types of data analysis provide a broad framework for understanding the overarching goals and purposes of data analysis, while data analysis techniques refer to a set of systematic approaches and methodologies used to analyze and interpret data for the purpose of extracting meaningful insights, identifying patterns, and making informed decisions. These techniques encompass a wide range of methods, tools, and processes designed to explore, transform, and model data, ultimately deriving valuable information from raw datasets. Some common data analytics techniques include:
- Exploratory Analysis: Used to understand the main characteristics of a dataset, employing visual methods like scatter plots and histograms.Think of it as using visual aids like scatter plots and histograms to get a clear picture of what’s going on in your data – it’s like looking at a map before starting a journey.
- Regression Analysis: Statistical method to understand relationships between dependent and independent variables, widely used in forecasting.This is like studying the connections between different aspects of your business. It helps predict future trends, almost like having a crystal ball for forecasting.
- Factor Analysis: Reduces a large number of variables into fewer factors, often applied in market research and customer segmentation.Imagine simplifying a big puzzle into smaller, more manageable pieces. This technique does just that, making it easier to understand what’s driving your business.
- Monte Carlo Simulation: Utilizes probability distributions and random sampling for estimating numerical results, commonly used in risk analysis.Ever played out different scenarios in your mind? This technique does that with numbers, helping you estimate outcomes and plan for uncertainties, much like preparing for different outcomes in a game.
- Cluster Analysis: Groups objects based on similarities, applied in market and image segmentation.Picture organizing your business into groups based on similarities, like putting similar products or customers together. It’s like creating strategic groups to better understand and cater to different needs.
- Cohort Analysis: Segments data into related groups for analysis, commonly used in marketing and customer lifecycle analysis.This is about breaking down your data into groups that have something in common. It’s like understanding how different sets of customers or events contribute to your overall success.
- Time Series Analysis: Statistical technique for analyzing trends in time series data, crucial for forecasting in various domains.Think of this as looking at the trends over time, much like tracking the performance of your business over different periods. It’s your business’s timeline analysis.
- Sentiment Analysis: Uses natural language processing to identify and extract subjective information, applied in social media monitoring and customer feedback analysis.It’s like having a tool to understand how people feel about your business by analyzing their comments or feedback online. It’s the emotional pulse of your brand in the digital world.
In conclusion, data analysis emerges as a transformative force in the contemporary business landscape. The process, from defining objectives to conveying insights, encompasses a series of crucial steps. The types of data analysis, ranging from descriptive to prescriptive, offer businesses a spectrum of insights, while various techniques enable a nuanced exploration of diverse datasets. As we navigate this data-driven era, mastering the art of data analysis becomes a critical skill, empowering businesses to adapt, innovate, and thrive in a dynamic world.
At Synogize, our commitment as a data analytics and AI consulting firm is to empower businesses to harness the full potential of their data. By leveraging our expertise, we guide clients through the intricacies of the data analysis process, ensuring a comprehensive understanding of their objectives.
Embark on a transformative journey with Synogize as your data analytics and AI consulting partner, and empower your business to not only navigate the complexities of data analysis but also unlock unparalleled insights for strategic decision-making and sustainable growth.