What is Data Lifecycle Management?
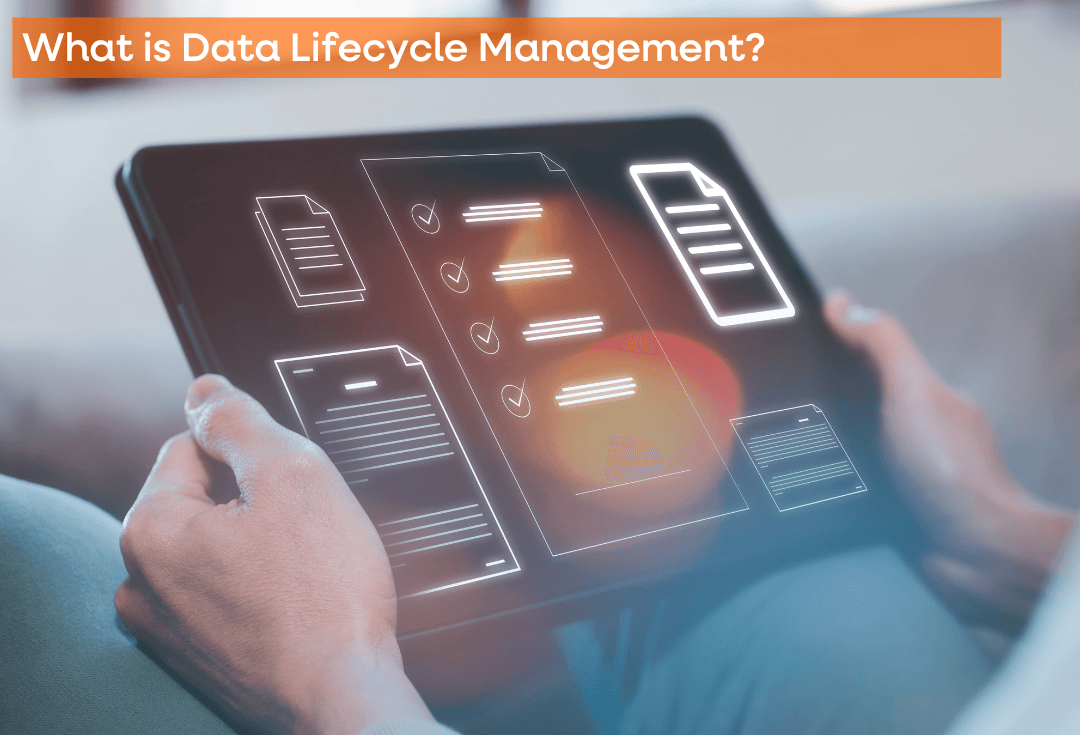
In today’s rapidly evolving digital landscape, Data Lifecycle Management (DLM) stands as a pivotal discipline that holds the key to unlocking the full potential of your data assets. This introductory blog aims to demystify the essence of DLM, offering invaluable insights into its phases and highlighting the multitude of benefits it can bring to modern enterprises.
What is Data Lifecycle Management?
Data Lifecycle Management embodies a strategic approach to overseeing the journey of data across its lifespan—from inception and collection to eventual archiving and disposal. It involves a series of carefully planned and designed stages, each governed by specific policies aimed at optimizing data’s utility while ensuring its security and compliance with prevailing regulations.
DLM goes beyond simple data handling, embracing a comprehensive framework that prioritizes data integrity, accessibility, and privacy throughout the data’s lifecycle.
Phases of Data Lifecycle Management
The journey of data through an organization is marked by several critical phases, each integral to the overarching goal of maximizing data value and minimizing associated risks:
1. Data Creation and Collection: Data can be either created or collected. Data creation in Data Lifecycle Management is the initial stage where raw data is generated within an organization. This phase involves shaping and structuring data with precision, focusing on format, quality, and relevance to business objectives.
Data collection on the other hand, is gathering data from diverse sources such as IoT devices, web applications, APIs, ERP systems, and different databases to name a few. Priority is given to collecting data that is not only high in quality but also relevant to business objectives, ensuring a solid foundation for subsequent stages.
The decisions made during this stage have a lasting impact on the entire data lifecycle, influencing downstream processes. Rigor in data creation enhances consistency, integrity, and usability, laying a solid foundation for subsequent stages such as storage, processing, analysis, and utilization. Overall, the importance of data creation lies in maximizing data value and minimizing risks throughout its lifecycle.
2. Data Storage & Processing: After collection, the data undergoes systematic storage and processing. This intricate process entails the meticulous organization of data based on its inherent nature—whether structured or unstructured—and the thoughtful selection of appropriate storage solutions tailored to each data type. For structured data, the utilization of relational databases is common, while unstructured data often finds its place in data lakes or non-relational databases.
An intriguing consideration emerges when data is moved for processing: its metadata does not accompany the transition. Metadata, containing critical information about the data’s origin, structure, and context, plays a pivotal role in understanding and utilizing the data effectively. Notably, when data is relocated, its metadata may not move seamlessly, leading to potential challenges in interpreting and managing the information.
This metadata oversight emphasizes the importance of a comprehensive understanding of the data’s context and structure, especially during transitions. The processing phase takes on additional significance as it involves crucial steps such as data encryption to fortify security measures, data wrangling for the transformation and cleansing of data into a more usable format, and adherence to regulatory standards like GDPR. This pivotal stage ensures that data is not only secure and compliant but also optimally prepared for analysis, facilitating insightful extraction and utilization in the broader spectrum of organizational decision-making.
3. Data Analysis and Visualization: With the data stored and processed, it moves into a phase of detailed analysis and visualization. This stage is where the true value of data is unlocked, as it is subjected to various analytical techniques—from basic exploratory data analysis to more complex processes like machine learning and statistical modelling. Visualization tools are employed to convert the insights derived from data into understandable and actionable visual formats, such as graphs, dashboards, and charts, facilitating informed decision-making and strategic planning.
4. Data Sharing and Usage: Data is now ready to be shared and utilized within the organization. At this point, DLM policies play a critical role in defining who can access the data and for what purpose, ensuring that its use aligns with business objectives without compromising its integrity or security. This phase is instrumental in leveraging data across different facets of the business, from enhancing operational efficiency to driving innovation and growth.
It’s important to note that when data is distributed, whether by sharing it with others or creating copies, it becomes challenging to maintain complete control over its entire lifecycle. Lifecycle management involves processes such as data organization, storage, processing, and security, among others.
When data is shared or copied, it may be utilized by individuals or systems outside the immediate control of the original data owner. This introduces the risk of unauthorized access, alterations, or usage that may not align with the initial intentions or policies set by the data owner. Additionally, tracking and managing the various copies of the data become more complex, making it difficult to enforce consistent policies throughout its lifecycle.
To mitigate these challenges, organizations often implement robust data governance and access control measures. These measures help maintain a level of control and security even after data has been shared or copied, ensuring that its usage remains aligned with organizational objectives and regulatory requirements.
5. Data Archival: Data not immediately needed but considered potentially useful for future use may be archived for long-term storage. This stage involves securely storing data that, while not needed for day-to-day operations, holds value for future reference, compliance, or regulatory purposes, adhering to predefined guidelines regarding the longevity and conditions of storage.
6. Data Deletion: The lifecycle concludes with the secure disposal (Commonly referred to as purge) of data that is deemed no longer necessary or relevant. This ensures the efficient management of storage resources and compliance with data retention policies, securely erasing data to protect against potential data breaches or misuse.
One noteworthy consideration when it comes to Data deletion, is the “right to be forgotten”; a legal concept that allows individuals to request the removal of their personal information from databases, often in compliance with data privacy regulations like GDPR. This right impacts data purging, as organizations must systematically delete relevant personal data when individuals exercise this right.
Compliance requires efficient mechanisms, clear policies, and procedures to handle requests promptly, as failure to do so can result in regulatory penalties and legal consequences for mishandling personal data. The “right to be forgotten” serves as a specific form of data purging, emphasizing the secure and responsible disposal of personal information once it is no longer necessary or requested for removal by the individual.
Benefits of Data Lifecycle Management
Implementing an effective DLM strategy confers several advantages, crucial for businesses aiming to thrive in the digital age:
1. Enhanced Process Efficiency: By maintaining data quality and relevance throughout its lifecycle, DLM facilitates process improvements, boosts operational efficiency, and enhances decision-making accuracy.
2. Cost Management: DLM helps in identifying data that has outlived its utility, allowing for cost-effective data storage solutions, and minimizing unnecessary expenditure on data maintenance.
3. Improved Data Usability and Accessibility: Consistent tagging and governance policies under DLM ensure that data remains organized and easily retrievable, thereby enhancing its usability for business operations.
4. Regulatory Compliance and Governance: With each industry subject to specific data retention and privacy standards, DLM aids in navigating these complexities, ensuring businesses remain compliant and mitigate the risk of legal repercussions.
In conclusion, as businesses continue to grapple with the challenges and opportunities presented by the digital data influx, the principles of Data Lifecycle Management stand out as a beacon of guidance. At Synogize, we believe in the importance of helping businesses navigate this complexity.
For companies looking to unlock the transformative potential of data, drive innovation, and secure a competitive advantage in the data-driven economy of 2024, embracing the structured approach of DLM becomes an essential stepping stone. By leveraging these principles, organizations can proactively manage their data, ensuring its relevance, compliance, and optimized utilization throughout its lifecycle.